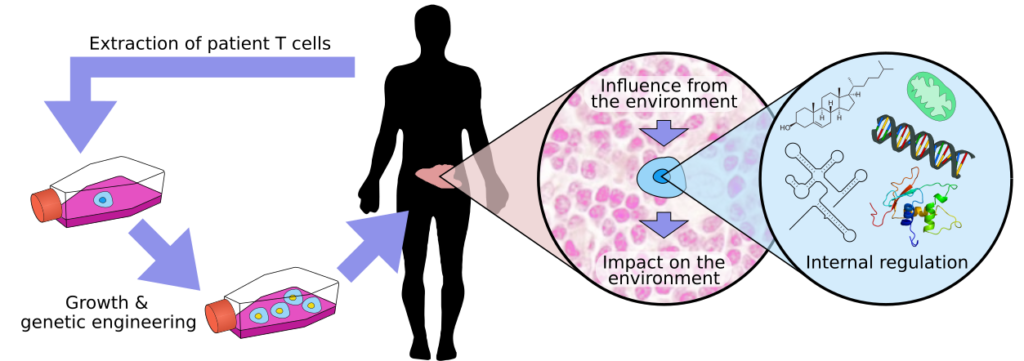
Note: We are actively recruiting. See “join us”
T cells are a key component of our adaptive immune system. We wish to figure out how T cells can be reprogrammed to better fight disease. Of particular interest are CAR T cells, which are taken from patients and genetically reprogrammed to cure cancer (figure above, left part). While we know ways of growing T cells in the lab, we understand the details surprisingly little. Poor manufacture can lead to brittle or misprogrammed T cells that don’t function well.
To know what reprogramming to do, we work on multiple levels. At the highest level we look at how T cells are affected by, and affect the environment. Cancer is infamous for adapting the tumour micro environment (TME) to prevent T cells from attacking it. We need to map a huge number of stimuli, from cytokines to metabolites, and see what interactions may happen.
To understand the impact of these stimuli, biology does unfortunately not offer an obvious place to look at. Rather, it seems spread across all the levels of regulation. To adress this huge space we use a combination of qualitative and quantitative analysis, developing new bioinformatics methods as required (Python, R, C, Java). We try to use modern “omics” protocols as much as possible, with a selection shown below. In addition, we have access to droplet single-cell equipment, and we develop our own improved wetlab protocols. Sequencing-based approaches is our speciality!
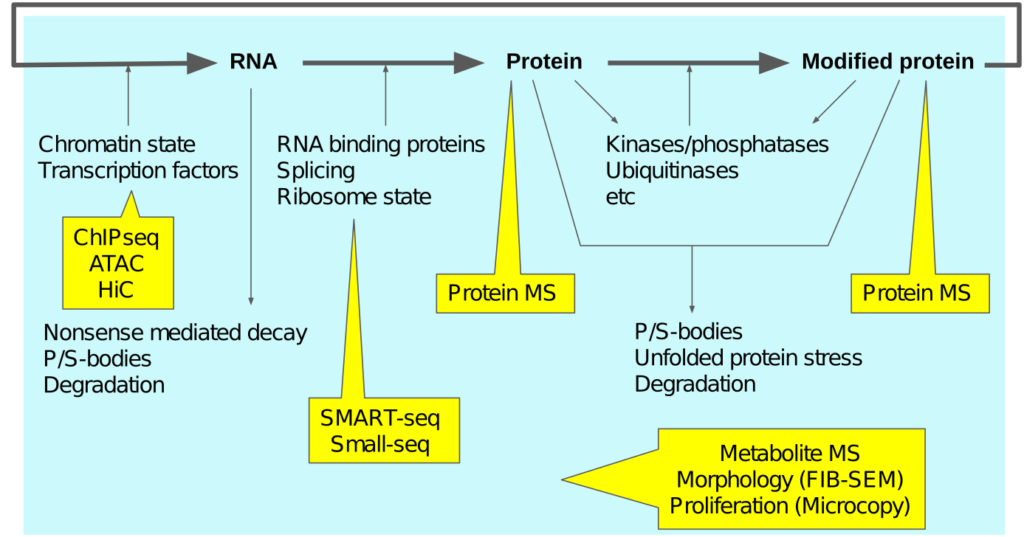
Our group has pioneered the use of CRISPR for finding genes regulating a certain process. To do this, a “cocktail” of viruses is added to the cells. Each virus regulates one target gene using CRISPR. By selecting the cells that behave in a certain way, and comparing to the ones that do not using sequencing, we can quickly find the genes we should be looking at. This way we can overcome plenty bias in which genes we study. It turns out we have had too much of a habit at looking at the same old genes, over and over!
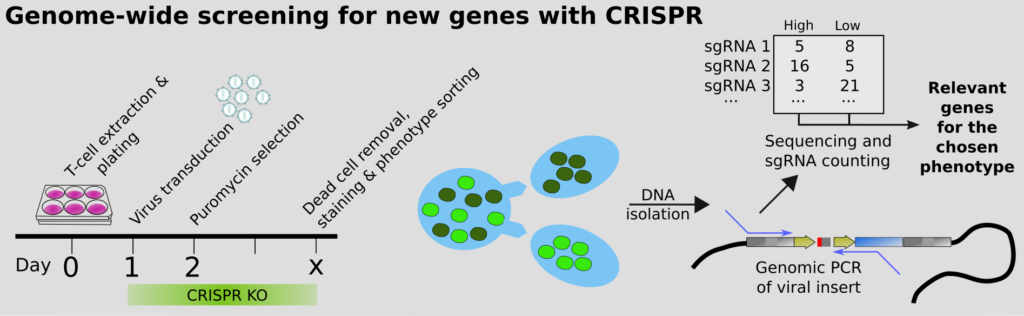
Once we know which genes are the main drivers of T cell behaviour in cancer, we are able to create sturdier T cells that can circumvent common tricks of the tumour. We can do use using viruses that deliver new genes (gene therapy), that in the case of CAR T cells contain a new anti-cancer receptor. From our screens and atlassing, we aim to find other regulatory mechanisms of the cells that we can reprogram. This can be done by including further “helper genes” in the viral construct.
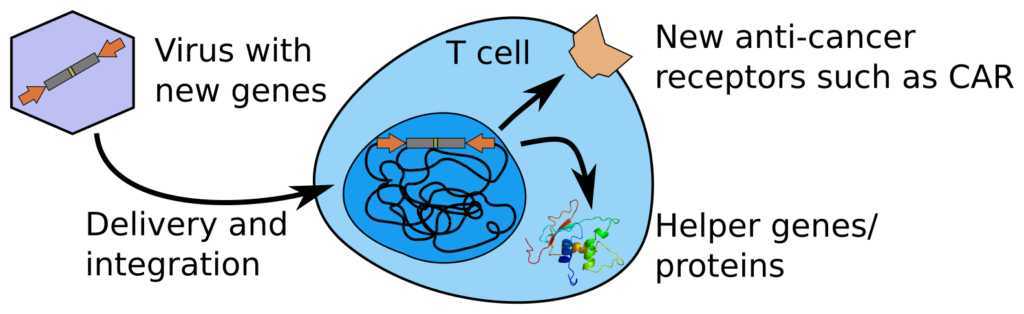